Tianqing Zhu
Title: Fairness in Machine Learning: Can Unlabeled Data Help to Reduce Discrimination?
Bio:
Tianqing Zhu is an Associate Professor with the School of Computer Science at the University of Technology Sydney, Australia. She is also the co-director of Centre for Cyber Security & Privacy at UTS. Tianqing Zhu received her B.Eng. degree and her M.Eng. degree from Wuhan University, China, in 2000 and 2004, respectively. She also holds a PhD in computer science from Deakin University, Australia (2014). Prior to joining UTS, she was a Lecturer with the School of Information Technology, Deakin University, from 2014 to 2018. Her research interests include privacy preserving, security in AI, and network security, and she has received more than 1.5 million AUD from the national fundings in the past five years.
Abstract:
A growing specter in the rise of machine learning is whether the decisions made by machine learning models are fair. While research is already underway to formalize a machine-learning concept of fairness and to design frameworks for building fair models with sacrifice in accuracy, most are geared toward either supervised or unsupervised learning. Yet two observations inspired us to wonder whether unlabled data might be useful to solve discrimination problems. First, previous study showed that increasing the size of the training set may lead to a better trade-off between fairness and accuracy. Second, the most powerful models today require an enormous of data to train which, in practical terms, is likely possible from a combination of labeled and unlabeled data. Hence, in this talk, we explore the possibility that a framework of fair semi-supervised learning in the pre-processing phase. A theoretical decomposition analysis of bias, variance and noise highlights the different sources of discrimination and the impact they have on fairness in semi-supervised learning.
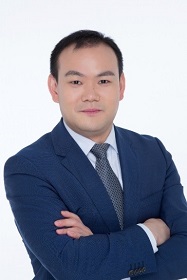
Jinjun Chen
Title: Effective Privacy Preservation in Blockchain
Bio:
Dr Jinjun Chen is a Professor from Swinburne University of Technology, Australia. He is Deputy Director of Swinburne Data Science Research Institute. He holds a PhD in Information Technology from Swinburne University of Technology, Australia. His research interests include data privacy and security, cloud computing, scalable data processing, data systems and related various research topics. His research results have been published in more than 200 papers in international journals and conferences, including various IEEE/ACM Transactions. He received various awards such as Editorial Excellence and Eminence Award of IEEE Transactions on Cloud Computing (2018), and UTS Vice-Chancellor’s Awards for Research Excellence Highly Commended (2014). He is currently an Associate Editor for ACM Computing Surveys, IEEE Transactions on Computers and IEEE Transactions on Sustainable Computing.
Abstract:
Blockchain presents many advantages such as decentralization and trust without credibility for underpinning various applications especially in finance. However, user privacy such as identity and contract sensitive data needs to be preserved properly. How to protect user privacy in blockchain comes to the picture and calls for effective solutions. Especially, we need to consider data utility when preserving user privacy. In this talk, I will illustrate my recent progress in this research challenge.